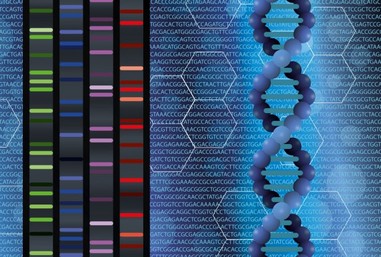
All hail to the computer scientists and their bioinformatics tools!
Let's say you are now ready to analyze your sequencing data. However, you have no idea what bioinformatics tools are available and which ones are the most convenient to use for your specific experiments. Well, if you didn't realize this before, it would be better for you to know that metagenomics is a field that is more suitable for the people that are savvy in computer languages or with experience in bioinformatics tools. But, don't let this get you down. There is still time to acquire skills in computer sciences, and you can even have fun during the process (for more information regarding computational tools that are useful for solving biological problems, visit this link).
Now, in relation to the metagenomic sequences, there are many options available that you can use for analyzing your data. The approach that you decide to use will depend greatly on your research objectives, and in the questions you want to answer. With this in mind, it is important that you understand some of the experimental and computational steps you have to cover during the acquisition and analysis of your data.
Below is a diagram that illustrates the experimental and computational steps that are necessary for a metagenomics study:
Let's say you are now ready to analyze your sequencing data. However, you have no idea what bioinformatics tools are available and which ones are the most convenient to use for your specific experiments. Well, if you didn't realize this before, it would be better for you to know that metagenomics is a field that is more suitable for the people that are savvy in computer languages or with experience in bioinformatics tools. But, don't let this get you down. There is still time to acquire skills in computer sciences, and you can even have fun during the process (for more information regarding computational tools that are useful for solving biological problems, visit this link).
Now, in relation to the metagenomic sequences, there are many options available that you can use for analyzing your data. The approach that you decide to use will depend greatly on your research objectives, and in the questions you want to answer. With this in mind, it is important that you understand some of the experimental and computational steps you have to cover during the acquisition and analysis of your data.
Below is a diagram that illustrates the experimental and computational steps that are necessary for a metagenomics study:
Houston, we have a solution!
In the brink of desperation, when nothing works and you have no clue whatsoever of what bioinformatics tools are available and which ones are the right ones to use for your experiment, there is still a chance for not letting anxiety take the best of you. Many bioinformaticians around the world are working in the development of better tools for analyzing genomic data. You can access some of these computational tools by clicking the items on the list below:
– DeconSeq
– MetaVelvet (de Novo assembler for metagenomic data)
– Celera Assembler
– Newbler
– Genovo
– Phrap
– MAP (Metagenomic Assembly Program)
– MetAMOS
– QUAST
– GeneMark
– GLIMMER
– PhymmBL
– MetaPhlAn
These and other tools are still available online and most are open-source. If you are interested in this topic and want more information about these computational approaches for analyzing your metagenomic data, I'll recommend that you take a look at the Metagenomics Informatics Challenges Workshop 2011 organized by the US Department of Energy Joint Genome Institute (JGI). In this series of talks, scientists discussed the capabilities and advantages of some of the computational tools that I mentioned in the list before. All the talks from the workshop are now available on YouTube. In the video below, you can watch the introductory talk to these workshops.
Good luck on your analysis!
*References:
(1) Angly et al. (2009) The GAAS metagenomic tool and its estimations of viral and microbial average genome size in four major biomes. PLoS Comput Biol. 5(12): e1000593.
(2) Hamady, M., and Knight, R. (2009) Microbial community profiling for human microbiome projects: Tools, techniques, and challenges. Genome Res. 19: 1141–1152.
(3) Kalyuzhnaya, M.G. et al. (2008) High-resolution metagenomics targets specific functional types in complex microbial communities. Nature Biotechnology 26(9): 1029–1034.
(4) Kunin, V. et al. (2008) A bioinformatician’s guide to metagenomics. Microbiol. Mol. Biol. Rev. 72(4): 557–578.
(5) Simon, C., and Daniel, R. (2011) Metagenomic analyses: past and future trends. Appl. Environ. Microbiol. 77(4): 1153–1161.
(6) Thomas et al. (2012) Metagenomics- a guide from sampling to data analysis. Microbial Informatics and Experimentation 2:3.
(7) Wooley, J.C., and Ye, Y. (2009) Metagenomics: facts and artifacts, and computational challenges. J Comput Sci Technol. 25(1): 71–81.
(8) Xu, J. (2006) Microbial ecology in the age of genomics and metagenomics: concepts, tools, and recent advances. Molecular Ecology 15: 1713–1731.
In the brink of desperation, when nothing works and you have no clue whatsoever of what bioinformatics tools are available and which ones are the right ones to use for your experiment, there is still a chance for not letting anxiety take the best of you. Many bioinformaticians around the world are working in the development of better tools for analyzing genomic data. You can access some of these computational tools by clicking the items on the list below:
- Metagenomic sequence pre-filtering
– DeconSeq
- Metagenomic-specific assembly
– MetaVelvet (de Novo assembler for metagenomic data)
– Celera Assembler
– Newbler
– Genovo
– Phrap
– MAP (Metagenomic Assembly Program)
– MetAMOS
- Assembly quality evaluation
– QUAST
- Metagenome analyzers (Gene prediction)
– GeneMark
– GLIMMER
- Metagenomic and/or profile species diversity analysis
– PhymmBL
– MetaPhlAn
These and other tools are still available online and most are open-source. If you are interested in this topic and want more information about these computational approaches for analyzing your metagenomic data, I'll recommend that you take a look at the Metagenomics Informatics Challenges Workshop 2011 organized by the US Department of Energy Joint Genome Institute (JGI). In this series of talks, scientists discussed the capabilities and advantages of some of the computational tools that I mentioned in the list before. All the talks from the workshop are now available on YouTube. In the video below, you can watch the introductory talk to these workshops.
Good luck on your analysis!
*References:
(1) Angly et al. (2009) The GAAS metagenomic tool and its estimations of viral and microbial average genome size in four major biomes. PLoS Comput Biol. 5(12): e1000593.
(2) Hamady, M., and Knight, R. (2009) Microbial community profiling for human microbiome projects: Tools, techniques, and challenges. Genome Res. 19: 1141–1152.
(3) Kalyuzhnaya, M.G. et al. (2008) High-resolution metagenomics targets specific functional types in complex microbial communities. Nature Biotechnology 26(9): 1029–1034.
(4) Kunin, V. et al. (2008) A bioinformatician’s guide to metagenomics. Microbiol. Mol. Biol. Rev. 72(4): 557–578.
(5) Simon, C., and Daniel, R. (2011) Metagenomic analyses: past and future trends. Appl. Environ. Microbiol. 77(4): 1153–1161.
(6) Thomas et al. (2012) Metagenomics- a guide from sampling to data analysis. Microbial Informatics and Experimentation 2:3.
(7) Wooley, J.C., and Ye, Y. (2009) Metagenomics: facts and artifacts, and computational challenges. J Comput Sci Technol. 25(1): 71–81.
(8) Xu, J. (2006) Microbial ecology in the age of genomics and metagenomics: concepts, tools, and recent advances. Molecular Ecology 15: 1713–1731.